Overview:
In this article, we delve into the methods of addressing the challenges in optimizing the Retrieval-Augmented Generation (RAG) pipeline as mentioned in the first part of our series. We emphasize the importance of measuring the performance of the RAG pipeline as a precursor to any optimization efforts. The article outlines effective strategies for evaluating and enhancing each component of the RAG pipeline.
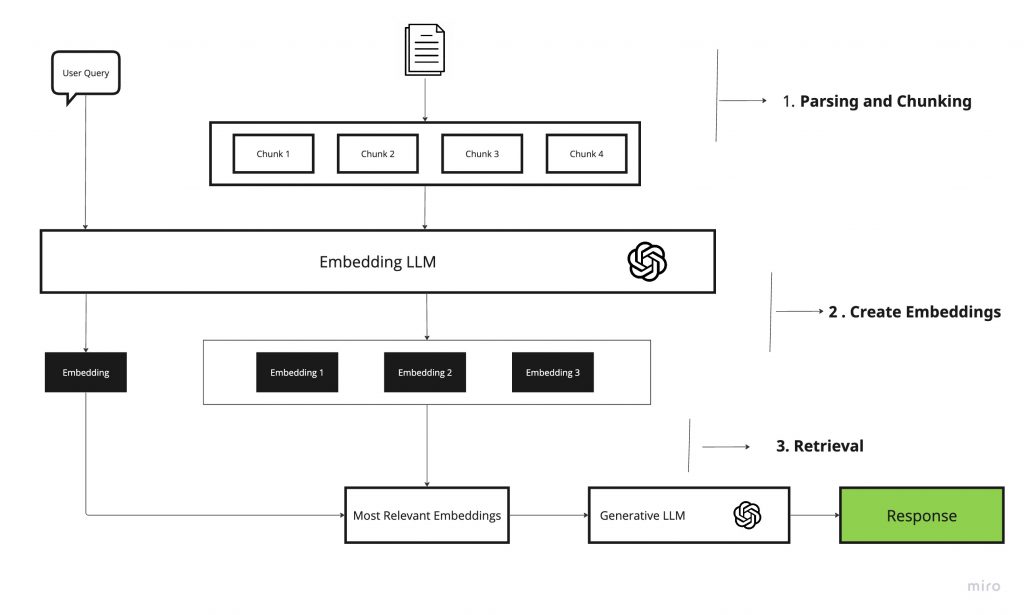
What can be done about the challenges?
- Data Management: Improving how data is chunked and stored is crucial. Rather than merely extracting raw text, storing more context is beneficial.
- Embeddings: Enhancing the representation of stored data chunks through optimized embeddings.
- Retrieval Methodologies: Advancing beyond basic top-k embedding lookups for more effective data retrieval.
- Synthesis Enhancement: Utilizing Large Language Models (LLMs) for more than just generating responses.
- Measurement and Evaluation: Establishing robust methods to measure performance is a fundamental step before proceeding with any optimizations.
Evaluation of the RAG Pipeline
The evaluation process is twofold:
- Evaluating each component in isolation
- Evaluating the pipeline end-to-end.
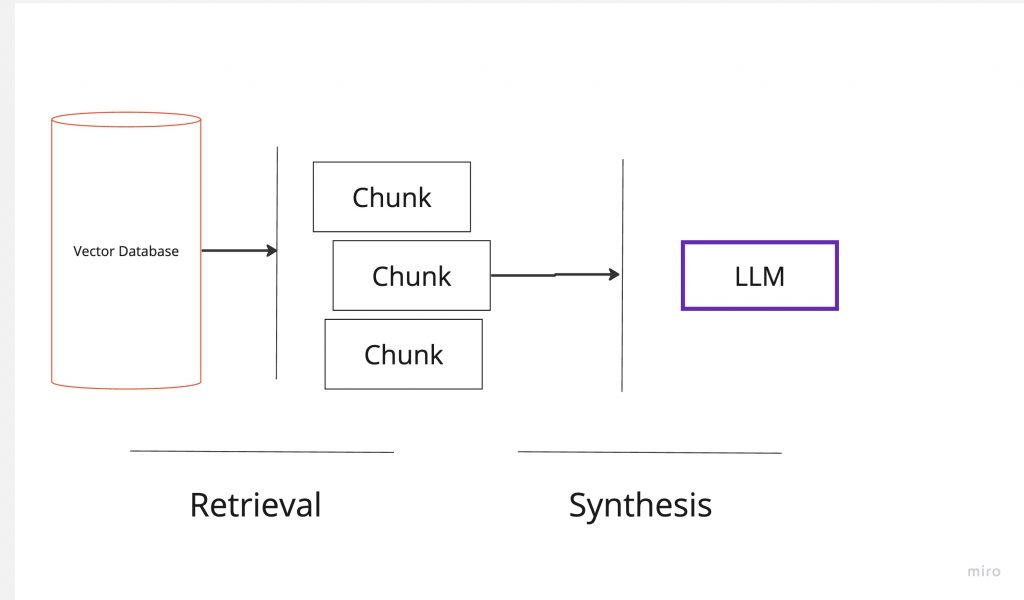
Evaluation in Isolation
- Retrieval: Ensuring the relevance of retrieved chunks to the input query.
- Synthesis: Verifying if the response generated aligns with the retrieved chunks.
Evaluation End-to-End
This involves assessing the final response to a given input by:
- Creating a dataset containing ‘user queries’ and corresponding ‘outputs’ (actual answers).
- Running the RAG pipeline for user queries and collecting evaluation metrics.
Currently, the field is evolving rapidly with various approaches for RAG evaluation frameworks emerging, such as the RAG Triad of metrics, ROUGE, ARES, BLEU, and RAGAs. In this article, we will discuss briefly about RAG triad and RAGAs. Both these models are known to evaluate RAG pipelines using LLMs rather than using human evals or ground truth evals.
RAG Triad of Metrics
The RAG Triad involves three tests: context relevance, groundedness, and answer relevance.
- Context Relevance: Ensuring the retrieved context is pertinent to the user query, utilizing LLMs for context relevance scoring.
- Groundedness: Separating the response into statements and verifying each against the retrieved context.
- Answer Relevance: Checking if the response aptly addresses the original question.
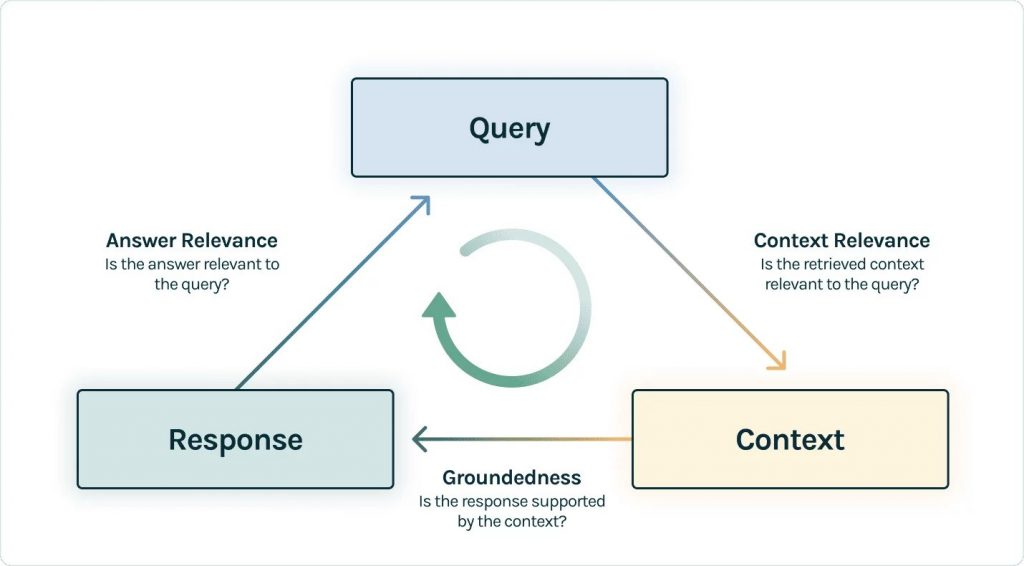
Context Relevance:
The initial step in any Retrieval-Augmented Generation (RAG) application is content retrieval, which is vital for ensuring the relevance of each context chunk to the input query. Any irrelevant context risks being incorporated into inaccurate answers. To assess this, we utilize the LLM to generate a context relevance score relative to the user’s query, applying a chain of thought approach for more transparent reasoning. We use this LLM reasoning capabilities for Groundedness and Answer relevancy metrics as well.
Example:
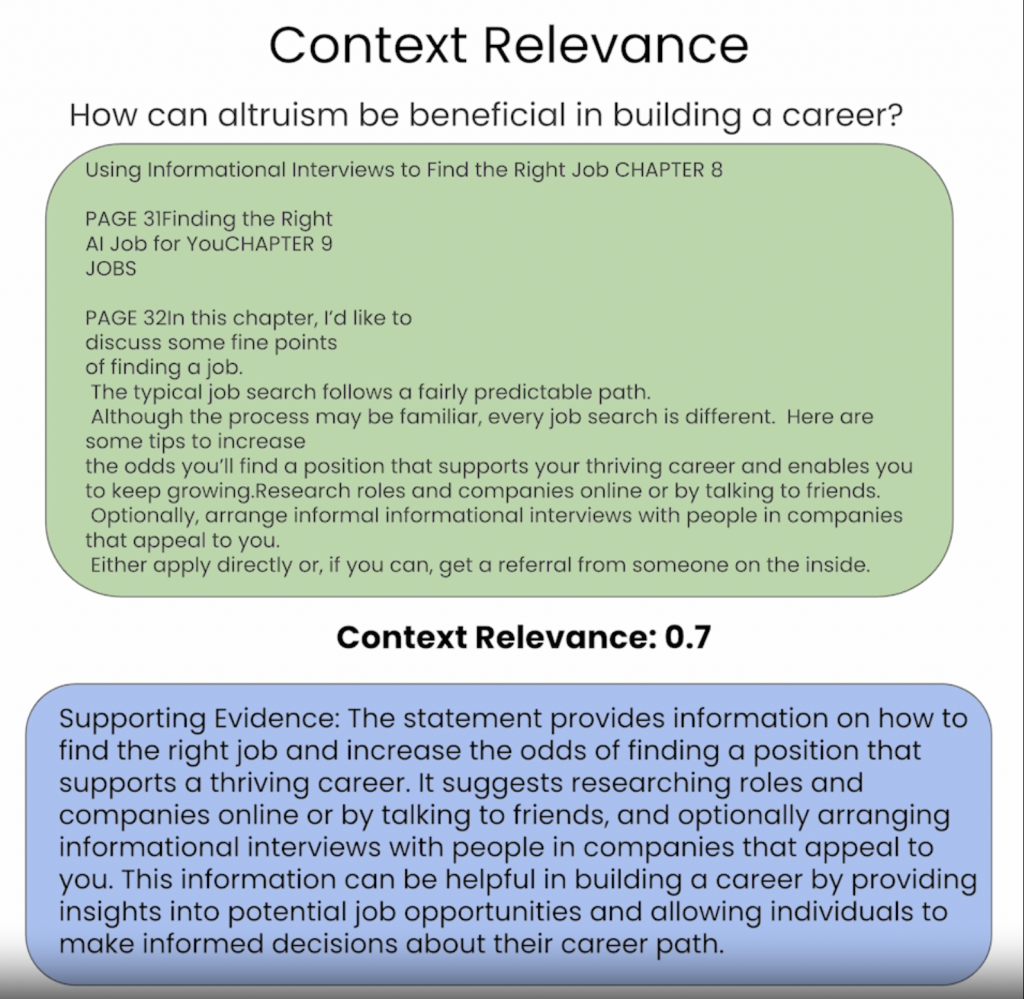
Groundedness:
Once the context is retrieved, a Language Model (LLM) crafts it into an answer. However, LLMs can sometimes deviate from the given facts, leading to embellished or overextended responses that seem correct but aren’t. To ensure our application’s groundedness, we dissect the response into distinct statements, and then independently verify the factual support for each within the retrieved context.
Answer Relevance:
Finally, our response must effectively address the user’s original query. We assess this by examining how relevant the final response is in relation to the user input, ensuring that it not only answers the question but does so in a manner that is directly applicable to the user’s needs.
By reaching satisfactory evaluations for this triad, we can make a nuanced statement about our RAG application’s correctness; our application is verified to be hallucination-free up to the limit of its knowledge base.
RAGAs
RAGAs (Retrieval-Augmented Generation Assessment) is a framework that aids in component-level evaluation of the RAG pipeline. It requires the user query (question), the RAG pipeline’s output (answer), the retrieved contexts, and ground truth answers.
Evaluation Metrics in RAGAs
- Context Precision and Recall: Assessing the relevance and completeness of the retrieved context.
- Faithfulness: Measuring the factual accuracy of the generated answer.
- Answer Relevancy: Evaluating the pertinence of the generated answer to the question.
- End-to-End Metrics: Including answer semantic similarity and answer correctness.
All metrics are scaled from 0 to 1, with higher values indicating better performance.
Conclusion
This article provides a comprehensive overview of evaluating and optimizing the RAG pipeline using LLMs. By effectively measuring each component and the pipeline as a whole, we can enhance the performance and reliability of RAG applications. The field is rapidly evolving, and staying abreast of new methodologies and frameworks is key to maintaining a cutting-edge RAG system. In the next part of this series, we will look into efficient parsing and chunking techniques.