Building Trust in AI: Ensuring Reliability and Accuracy in AI Assistants
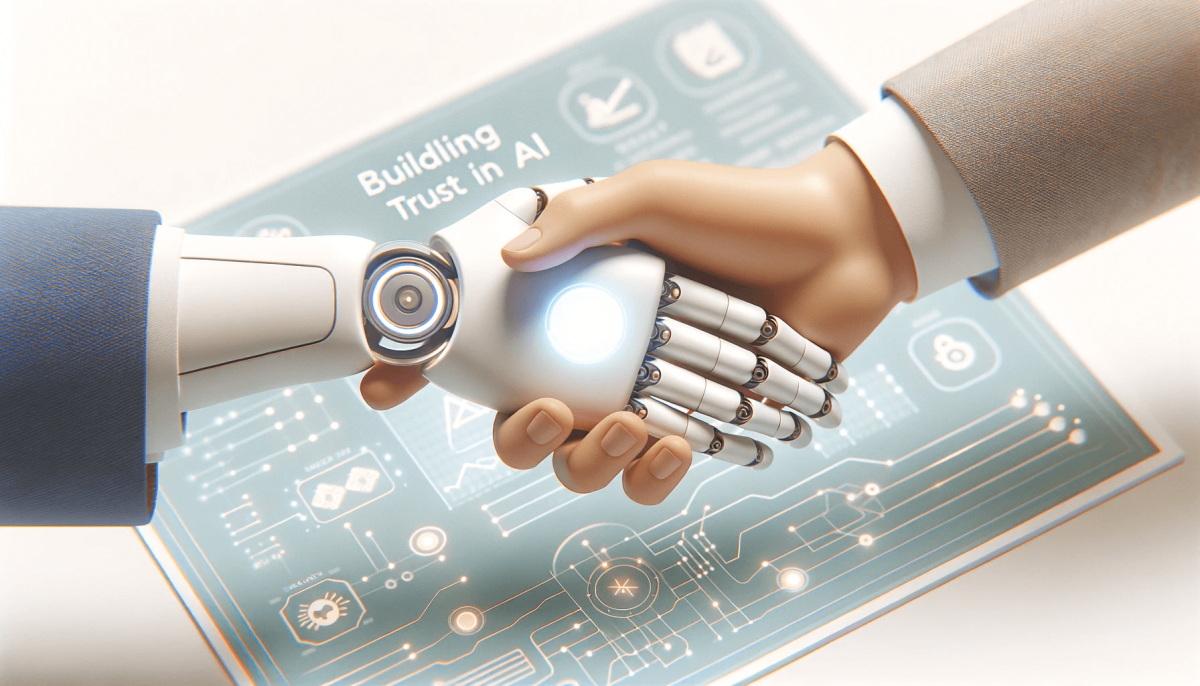
Building Trust in AI: Ensuring Reliability and Accuracy in AI Assistants
Introduction
In today’s technologically advanced era, the prevalence of artificial intelligence (AI) across various sectors has been nothing short of transformative. AI assistants, particularly those powered by large language models (LLMs), are reshaping industries such as customer service, healthcare, and finance. Their role in assisting with decision-making processes and influencing our daily activities is becoming increasingly pronounced. As AI becomes more embedded in our lives, the crucial question of trust arises: How do we ensure and enhance the trustworthiness of AI-generated content, especially in the realm of text generation? This article seeks to address this question, exploring strategies to develop AI assistants that are not only intelligent but also reliable, accurate, and trusted by their users.
Understanding Trust in AI-Generated Content
Trust in AI-generated content, especially in the context of text produced by LLMs, is a complex issue. It extends beyond mere technical accuracy, encompassing aspects such as competence, reliability, and ethical integrity. Trust is a critical component in AI interactions, influencing user acceptance and reliance on these technologies. In AI assistants, where decisions based on AI-generated text can significantly impact various aspects of both personal and professional life, establishing trust is paramount.
Challenges to Trust in AI
Inaccuracies and Variability: One of the primary challenges in AI-generated content is the presence of inaccuracies and variability. For example, in the financial sector, inconsistent AI advice could lead to poor investment decisions, while in education, inaccurate information could misinform students. Variability in AI responses can also result in an inconsistent user experience, undermining the user’s confidence in the system.
Biases in Data: Another critical challenge is the presence of biases in the training data of AI systems. These biases can manifest in various applications, such as language processing and facial recognition, leading to skewed and often unfair outcomes. For example, AI systems might exhibit racial bias in predictive policing or gender bias in job recruitment algorithms, reflecting the biases present in their training data.
Real-World Consequences: The consequences of AI errors can be significant, particularly in sensitive areas such as healthcare and law enforcement. Misinterpretations or biases in AI can lead to incorrect medical diagnoses or unjust legal decisions, showcasing the critical need for reliable and unbiased AI-generated content.
Strategies for Enhancing Trustworthiness
Advanced Data Validation: To combat biases and inaccuracies, advanced data validation is essential. This involves using sophisticated methods and technologies to ensure the data feeding into AI models is accurate, representative, and free from biases. In the realm of LLMs, this means creating diverse and inclusive training datasets, which have been shown to significantly reduce biases and improve the performance of AI systems.
Iterative Training Processes: Updating AI models with new, diverse datasets is crucial in maintaining their accuracy and relevance. This iterative process helps AI systems stay current with evolving language usage and societal norms, reducing the likelihood of outdated or biased outputs.
Error-Checking Algorithms: Incorporating algorithms that can autonomously detect and correct errors in AI-generated text is vital for maintaining the reliability of these systems. Such algorithms play a crucial role in ensuring the accuracy and consistency of the content generated by AI assistants.
Continuous Learning: The concept of continuous learning in machine learning models, particularly in text generation, is vital for maintaining their accuracy over time. This approach allows AI systems to adapt and evolve with new information, maintaining their relevance and accuracy in a rapidly changing world.
Transparency and User Control
Transparency in the decision-making processes of AI systems is essential for building trust. Users need to understand how AI assistants reach their conclusions. Implementing user control mechanisms, such as customization options and feedback systems, not only enhances trust but also improves the accuracy of AI systems. For instance, allowing users to understand and, if necessary, correct the reasoning behind an AI-generated piece of text can lead to better outcomes and greater user satisfaction.
Measuring and Monitoring AI Performance
Ongoing monitoring and measurement of AI performance are critical in building and maintaining trust. Employing robust metrics and analytics to track the accuracy and reliability of AI systems enables continuous improvements. Monitoring user interactions with AI-generated content, such as the frequency and nature of corrections or queries, provides valuable insights into areas where the AI needs refinement.
Future Directions and Innovations
Explainable AI (XAI): XAI focuses on making AI decisions more transparent and understandable to humans. By demystifying how AI systems reach their conclusions, XAI can significantly enhance trust in these technologies.
Human-AI Collaboration: The future of AI lies in a collaborative approach, where human intuition and expertise are combined with AI’s computational efficiency. This synergy can optimize decision-making processes, leveraging the unique strengths of both human and artificial intelligence.
Ethical AI Frameworks: The development and adherence to ethical guidelines are crucial in ensuring that AI systems are not only accurate but also fair and unbiased. These frameworks guide the responsible development and deployment of AI, ensuring that it serves the greater good.
AI Auditing: Independent audits of AI systems are essential for ensuring adherence to standards and building public trust. Regular audits by external bodies can verify the accuracy, fairness, and reliability of AI systems, holding them accountable to established norms and expectations.
Conclusion
Building trust in AI, particularly in text-generative AI assistants, is a complex and multifaceted challenge. It requires a combination of technical solutions, ethical considerations, and continuous user engagement. As AI assistants become more integrated into our daily lives and business processes, the importance of trust in these systems cannot be overstated. By addressing these challenges and embracing innovative solutions, we can pave the way for AI assistants that are not only intelligent but also reliable, accurate, and trustworthy. The future of AI trustworthiness is promising, with ongoing advancements in technology and a growing focus on ethical and responsible AI development. As we continue to evolve these technologies, the emphasis on building and maintaining trust will remain a cornerstone of their success and acceptance in society.