The Story of AI: Key Moments in History
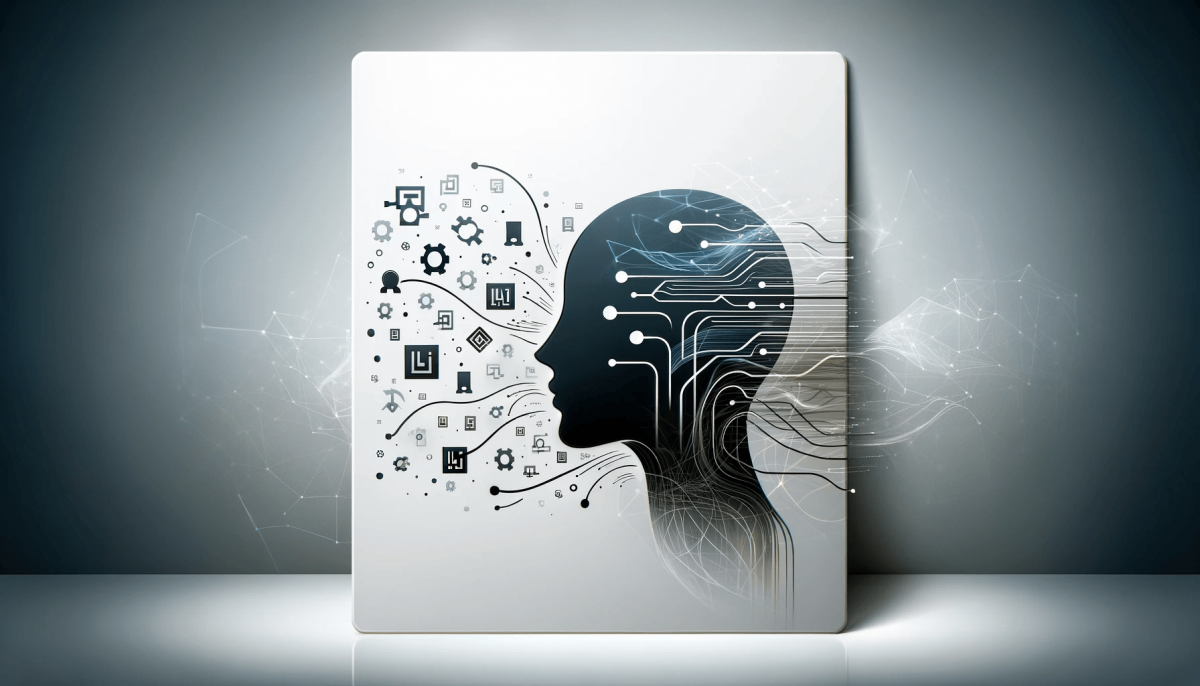
The Story of AI: Key Moments in History
Introduction
In the fast-evolving landscape of Artificial Intelligence (AI), we stand at a significant juncture, looking ahead at the tantalizing prospect of Artificial General Intelligence (AGI) – a frontier where machines can perform any intellectual task that a human being can. The journey to this point has been one of extraordinary innovation, resilience, and breakthroughs, each pushing the boundaries of what we thought possible. This article aims to take you through this remarkable journey, focusing on key research papers and discoveries that have shaped the field of AI, bringing us ever closer to the dream of AGI.
Our story is not just about technology; it’s about human ingenuity and vision. It’s a narrative of challenges identified, addressed, and sometimes left open for the next brilliant mind to solve. So, let’s embark on this journey through time, understanding how each milestone has contributed to the evolving landscape of AI and what challenges were left for the next leap forward.
“Perceptrons” by Marvin Minsky and Seymour Papert (1969)
Our story begins in 1969, with the seminal work “Perceptrons” by Marvin Minsky and Seymour Papert. This book, often misunderstood, played a paradoxical role in AI’s history. While it was critical of the early neural networks, known as perceptrons, for their limitations in handling complex patterns, it inadvertently set the stage for future breakthroughs. Minsky and Papert pointed out that these early networks could not solve problems requiring the understanding of hierarchical structures or deep patterns in data, a significant limitation of the AI of their time.
But here’s the catch – in highlighting these limitations, “Perceptrons” also outlined the exact challenges that needed to be overcome. It set a clear direction for future research: How can we develop networks capable of more complexity and depth? This question lingered in the air, a challenge awaiting those daring enough to take it on.
The main challenge left unresolved by Minsky and Papert was the need for neural networks capable of handling a greater depth of complexity. This challenge would echo through the next decades, inspiring researchers to push the boundaries of neural network design.
“Learning representations by back-propagating errors” (1986)
Fast forward to 1986, and we arrive at a significant breakthrough: the concept of backpropagation, introduced in the paper “Learning representations by back-propagating errors” by David E. Rumelhart, Geoffrey E. Hinton, and Ronald J. Williams. This was the answer to Minsky and Papert’s challenge. Backpropagation is a way for AI to learn from its mistakes, akin to human learning. When an AI makes an error, backpropagation allows it to adjust its internal parameters, essentially learning what it did wrong and how to do it better next time.
This method enabled the development of more complex and deeper neural networks, which could learn and adapt in ways previously impossible. It was a giant leap forward, allowing AI to tackle more complex tasks and opening up a new realm of possibilities.
However, this breakthrough also left a new challenge in its wake: despite the improved learning capabilities, neural networks still struggled with certain tasks, particularly in the realms of image recognition and language processing. The world of AI was poised for the next big leap – one that would enable machines to ‘see’ and ‘understand’ in ways akin to humans.
“ImageNet Classification with Deep Convolutional Neural Networks” (2012)
The year 2012 marked a watershed moment in AI with the introduction of deep convolutional neural networks (CNNs), primarily through the work “ImageNet Classification with Deep Convolutional Neural Networks” by Alex Krizhevsky, Ilya Sutskever, and Geoffrey Hinton. This paper demonstrated a stunning improvement in the ability of AI to recognize and classify images in the ImageNet challenge, a large-scale visual recognition competition.
The innovation of CNNs can be likened to giving AI a rudimentary form of ‘sight’. These networks were designed to process and recognize patterns in images in a way similar to the human visual cortex. This breakthrough was not just about better image recognition; it was a fundamental shift in how AI could process complex, unstructured data like pictures, laying the groundwork for advancements in computer vision applications ranging from medical diagnostics to autonomous vehicles.
However, this success in vision posed a new question: could AI achieve similar mastery in understanding and processing language? While strides were being made in image recognition, the complexity of human language remained a largely unconquered domain.
“Playing Atari with Deep Reinforcement Learning” (2013)
Enter the realm of DeepMind’s “Playing Atari with Deep Reinforcement Learning” in 2013. This paper was groundbreaking, showcasing an AI that could learn to play Atari video games at a superhuman level. This wasn’t just about gaming; it was a demonstration of reinforcement learning, where AI learns optimal behaviors through trial and error, rewarded for positive actions – a learning process akin to that of humans and animals.
This development was crucial. It showed that AI could not only learn from large datasets but also from its own experiences, adapting and optimizing its strategies in dynamic environments. The challenge that emerged from this was clear: how do we create AI systems that can generalize this learning ability, applying knowledge and skills across various domains and not just in the specific environment they were trained in?
“Attention Is All You Need” (2017)
The paper “Attention Is All You Need” in 2017 marked a significant leap in natural language processing. This work introduced the Transformer model, which used a novel mechanism called ‘Attention’ to process sequences of data (like sentences in language). The beauty of this approach was in its simplicity and power – the Transformer could focus on different parts of the input data at different times, mimicking how human attention works, leading to more efficient and effective language models.
This innovation was monumental in enabling AI to understand and generate human language with unprecedented coherence and fluency. It opened the door for models that could handle tasks ranging from translation to content creation with a level of sophistication that was previously unattainable. The ensuing challenge was now to build AI systems capable of not just understanding language but reasoning and thinking across diverse domains – a step closer to AGI.
“Mastering Chess and Shogi by Self-Play with a General Reinforcement Learning Algorithm” (AlphaZero) (2017)
The year 2017 also saw the rise of AlphaZero, a system developed by DeepMind that mastered the games of Chess and Shogi through self-play. AlphaZero’s approach was revolutionary. Unlike previous AI that relied on vast databases of human games, AlphaZero learned to play these games at a superhuman level by playing against itself, starting from scratch with no prior knowledge except the game rules.
This approach demonstrated the power of self-learning and generalization in AI. AlphaZero’s ability to learn and master complex games without human intervention was a glimpse into the potential of AI systems that could discover and learn from the underlying structures of various problems. The question it left in its wake was how to develop AI that could apply this self-learning ability in real-world scenarios, beyond the structured environment of games.
“BERT: Pre-training of Deep Bidirectional Transformers for Language Understanding” (2018)
In 2018, the AI world witnessed another milestone with the introduction of BERT (Bidirectional Encoder Representations from Transformers) by researchers at Google. BERT represented a significant step forward in natural language understanding. Unlike previous models, BERT was designed to understand the context of a word in a sentence – capturing the nuances and complexities of human language more effectively than ever before.
BERT’s innovation lay in its ability to process and interpret the meaning of words in relation to all the other words in a sentence, rather than one direction at a time. This advancement dramatically improved the performance of AI in a range of language tasks, from sentiment analysis to question answering. The challenge following BERT’s success was creating AI that could not only understand language but also exhibit broader cognitive abilities like reasoning, problem-solving, and learning in a more human-like manner.
Conclusion
As we trace the arc of AI’s evolution through these pivotal research papers, we witness a journey of remarkable ingenuity and relentless pursuit of understanding. Each breakthrough brought us closer to the dream of AGI, while also unveiling new challenges and frontiers to explore. Today, as we stand on the shoulders of these monumental achievements, the path to AGI seems not just a possibility but an inevitable next step in this incredible journey of discovery.
In the end, the story of AI is one of human aspiration – a testament to our quest for knowledge and our desire to expand the boundaries of what is possible. As we look ahead, the future of AI promises not just technological transformation but a new era of innovation and understanding, reshaping our world in ways we are just beginning to imagine.