5 easy steps to train your chatbot in 2024
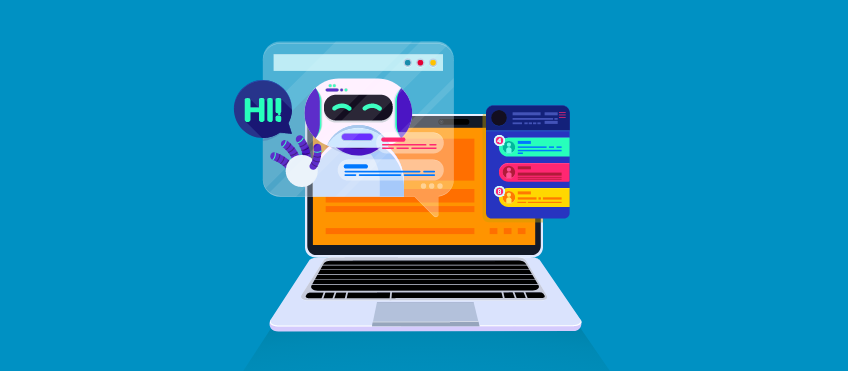
5 easy steps to train your chatbot in 2024
Today, most businesses have switched to digital platforms and they have started communicating with their customers via different communication sources.
Social media, emails, chatbots, and everything that can be named.
A chatbot is the frontline brand representative of your company. It is also the first point of contact with your customer, and probably your first impression. Being the first impression, the chatbot must perform well and relevantly. For this purpose, you must train your chatbot accordingly.
It is unpredictable to determine the kind of queries a human might come across. Someday, a query might be, find me a perfect blue-colored dress or find me something in pink. Now, these queries might sound logical to a human being but to a chatbot, this might sound confusing sometimes.
In this article, we have covered all the essential pointers you need to keep in mind while training your chatbot:
- Defining your target persona
- Categorization of customer queries
- Creating and training chatbots with datasets
- Asking users to test the chatbot
- Improvising the chatbot
Now, let’s study each of this one by one.
Defining your target persona:
The target persona is the foundation of chatbot development. The training stage comes later. Every time you make a decision, you’ll hit back to the target persona.
You need to start chatbot development by identifying the type of your audience. Give your chatbot a voice, a tone, and a relevant flow. Other parameters like vocabulary matter too. Adding to that, you should also consider age, gender, location, language, income, profession, industry, behavior, interests, and most significantly, the challenges that are likely to occur. Later, you can form some demo personas. Also, you don’t need to build only one target persona. You can have multiple, to begin with.
Later, you can analyze the data and form some sample questions based on the persona. One quick way to define your target persona can be by connecting with your customer support team. Since they’re the people who handle customer queries daily, they are in a better position to give you a rough understanding of the kind of queries the chatbot might come across.
Categorization of customer queries:
Categorization saves a stitch in time. The types of queries can be categorized into different categories like informational, regarding the product, tracking, services, or any grievances. The simplest thing anyone can follow is by connecting with the support team or sales reps. There exists your gold mine of queries. Often, people call up the support team in expectation of getting their queries solved. Also, the emails like @info or @contact can be dug up too.
The best resource to find common customer queries would be the customer service center of the organization. For example, if you find several queries like “How to use this?”, “How to operate this product?”, “How to fix an XYZ error?”, you can group all such queries under the category of “Product Info”.
Create and train your chatbot with datasets:
Datasets are like knowledge stacks for a chatbot. The more they are trained with one, the more efficient they become. Once you group up all your queries, it becomes necessary to either find alternatives to these are find a wide range of answers for the same. A chatbot training involves much than just training. Right from collecting to analyzing data, and formulating the next steps, a lot goes into the process.
However, there are two easy steps you can follow:
Training your chatbot with the available data:
As we mentioned above how you can collect data from the customer support team, the same can be used to come up with a certain dataset that includes commonly asked questions. A little brainstorming about phrases or specific keywords you would ask a chatbot can help build a dataset. Think of all the ways or variations you’d ask a question to a chatbot.
Training your chatbot with ready-to-use datasets:
Another alternative can be to train your chatbot using pre-made or ready-to-use datasets.
Here are a few categories and some sources of datasets that can be handy:
1) Q & A Datasets:
- Question and Answer Dataset: This collection includes all the Wikipedia articles, manually-generated factoid questions from them, and manually-generated answers to these questions, for use in academic research.
- The WikiQA Corpus: This corpus is a publicly available dataset whose source is Bing query logs. Each of its queries has a probable solution linked to Wikipedia.
2) Customers Support Datasets:
- Ubuntu Dialog Corpus: Ubuntu Dialog Corpus includes almost one million two-person conversations extracted from the Ubuntu chat logs. These conversations were used to receive technical support for various Ubuntu-related problems. The full dataset contains 930,000 dialogues and over 100,000,000 words.
- Customer Support on Twitter: This corpus on Kaggle includes replies from the biggest brands on Twitter.
3) Dialogue Datasets:
- Cornell Movie-Dialogs Corpus: With a large metadata-rich collection of fictional conversations extracted from raw movie scripts, this corpus includes 220,579 conversational exchanges between 10,292 pairs of movie characters involving 9,035 characters from 617 movies!
- Santa Barbara Corpus of Spoken American English: This dataset includes approximately 249,000 words of transcription, audio, and timestamps at the level of individual voice modulation units.
- The NPS Chat Corpus: This corpus consists of 10,567 posts out of approximately 500,000 posts gathered from various online chat services following their terms of service.
4) Multilingual Chatbot Training Datasets:
- NUS Corpus: This corpus was created to normalize and standardize social media texts. It is built by randomly selecting 2,000 messages from the NUS English SMS corpus and then translated them into formal Chinese.
Ask real users to test the chatbot:
Ask your staff or your real customers to test the chatbot. When a human interacts with a chatbot, that is when you realize where the loopholes are.
In the initial beta testing phase, you can ask your co-workers to test the bot.
Remember, your co-workers may have a completely different perspective, and they may be familiar with different terminology, vocabulary, information, etc. They are not your chatbot audience. Hence, their opinions and queries may vary from the ones that are desired.
Another way to go is by encouraging your loyal customers in the process. Since customers may ask questions that can be listed down as potential queries, it would be a win-win situation for you.
You can also hire QA from various platforms like Fiverr, Upwork, etc. Another way to test your chatbot would be using some platforms like Botium, Zypnos, or qbox.ai platforms to test the bot.
There are also a few freelancers whom you can hire for this purpose.
Keep on improvising your chatbot:
- In the initial days, the chatbot might need human agent help to deal with the situations. As a freshly launched chatbot, it might come across certain queries completely new and unfamiliar. Also, the more it is trained, the better it gets.
- Keep analyzing the kind of interactions your chatbot is having with visitors. Look for all the underperforming answers and try to rephrase them.
- Look for all the errors and loopholes in the chat flow. Check the analytics for the performance of the chatbot. ChatGen’s dynamic dashboard provides in-depth research about the chatbot performance like at which point of conversation did the users drop or leave the chat, etc.
- Also, do not forget to support your chatbot in the future as well.